Introduction
Decision Support Systems
Decision Support Systems (DSS) are a specific class of computerized information system that supports decision-making activities. DSS are interactive computer-based systems and subsystems intended to help decision makers use communications technologies, data, documents, knowledge and/or models to identify and solve problems and make decisions. Five more specific DSS types include:
Communications-driven DSS
Data-driven DSS
Document-driven DSS
Knowledge-driven DSS
Model-driven DSS
OLAP
The term, of course, stands for ‘On-Line Analytical Processing’.
OLAP is a method that allows users to extract data from a database and turn it into information that can be used for making business decisions. The OLAP process is an interactive one, and it allows organizations to view their data strategically so that they can make predictions about the future in addition to understanding their history.
During the last ten years, a significant percentage of corporate data has migrated to relational databases. Relational databases have been used heavily in the areas of operations and control, with a particular emphasis on transaction processing (for example, manufacturing process control, brokerage trading). To be successful in this arena, relational database vendors place a premium on the highly efficient execution of a large number of small transactions and near fault tolerant availability of data.
While OLAP systems have the ability to answer "who?" and "what?" questions, it is their ability to answer "what if?" and "why?" that sets them apart from Data Warehouses. OLAP enables decision-making about future actions. A typical OLAP calculation is more complex than simply summing data, for example: "What would be the effect on soft drink costs to distributors if syrup prices went up by $.10/gallon and transportation costs went down by $.05/mile?"
OLAP and Data Warehouses are complementary. A Data Warehouse stores and manages data. OLAP transforms Data Warehouse data into strategic information. OLAP ranges from basic navigation and browsing (often known as "slice and dice"), to calculations, to more serious analyses such as time series and complex modeling. As decision-makers exercise more advanced OLAP capabilities, they move from data access to information to knowledge.
The distinction between a data warehouse and data mart is scope. The warehouse typically stores enterprise-wide data, whereas a data mart stores information for a smaller entity—for example, an individual hospital instead of the entire healthcare company. While industry watchers have recently been focusing on Java and the Web, many organizations have been accumulating massive amounts of data in data warehouses. Now those organizations are promoting a marriage of Web and data-warehousing technologies.
A typical internet/intranet data warehouse scenario is a client/server architecture with Web OLAP clients that connect to an OLAP server tied to an SQL database. Your users submit queries that return a multidimensional data cube as the query result. Users will often want to perform various types of analysis using the data cube—a process that is called "slicing and dicing." Depending on the OLAP engine, the data cube might be cached at the server or downloaded to the client and cached for offline analysis. The market is starting to see the emergence of server-side OLAP engines, but there is more Java client software for OLAP than server software.
Databases and queries used for OLAP are quite different from those used by applications such as brokering stocks or processing orders. When designing databases and queries for a DSS application, your guidelines are fundamentally different from a developer using an operational data store for online transaction processing (OLTP). A Web shopping cart, for example, will have high numbers of users running short-lived queries against an OLTP database. A decision-support application will often serve a small number of users executing long-lived queries. An operational store, such as an orders database, typically contains information for a 60- to 90-day period but a data warehouse often contains two years or more of historical data. The operational store contains measurements and facts that paint an accurate picture of the current period or slice in time, but a data warehouse represents multiple time slices and measurements that describe years of operation. This enables you to aggregate data, analyze variances, detect trends, and run decision-support queries with data pivots and drill-downs.
The accumulation of years of data can produce massive databases. A large book distributor's data warehouse includes a core table with 200 million rows. A data warehouse with two years of frequent-flier information is likely to have a flight history table with 60 million rows using four GBs of disk storage. Large enterprises often require massively parallel hardware and very large decision-support databases. Sears Roebuck and Company uses the NCR Teradata DBMS to manage a database that is 4,630 gigabytes (4.63 terabytes).
Decision Support with Schemas
One of the primary factors in the success of a data warehouse project is database design. Designers creating databases for OLTP applications often use data-modeling techniques intended to remove data redundancy. These techniques produce designs that are well suited for OLTP performance, but are not optimal for OLAP. The end users who comprise the decision-support audience also not easily understand them.To produce a more intuitive design that delivers good performance, data warehouse designers often use a dimensional model and star schema for SQL databases. The star schema uses a fact table to store numerical measurements about the subject of interest, such as shipments, sales, purchases, subscriptions, car rentals, and so on. It uses dimension tables to store attributes that are textual and discrete. For example, a sales-fact table will often be linked with a product-dimension table to produce information about product sales. If the schema includes a time dimension table, you can run queries about product sales over time. If you add a region dimension, you can run queries about sales by product and region.
Figure 1 illustrates a star schema having a single fact table and multiple dimension tables.
Because star schemas have become so widespread for dimensional data warehouses, some DBMS vendors have modified their SQL optimizers to recognize star schemas. The optimizers for NCR Teradata, IBM DB2 Universal Database, Oracle 8, and Microsoft SQL Server 7.0 include logic to generate execution plans for queries against star schemas. There is an ongoing debate about whether to use a star schema, or simply tables that are normalized to third normal form (3NF), when designing databases for relational OLAP (ROLAP) applications. In either case, your Java code uses an SQL API and submits SQL.
OLAP queries operate on multidimensional data sets or data cubes, whether or not they use a star schema.
Gleaning the Cube OLAP programs operate with multidimensional data sets known as cubes. The elements of this cube represent measurements for a unique combination of product, sales, and time. (Figure 2)
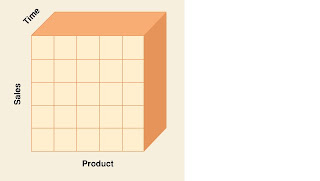
Figure 2 is an example of a cube having a product, sales, and time dimension.
Six Tips for Better OLAP Designs
1. Don't blindly jump into a project. Implement some pilot projects before building a large data warehouse.
2. Check your query timing. Java's threaded architecture and the cancel() method of the JDBC statement object enable you to include logic for aborting long-running queries. However, remember decision-support queries often run longer than OLTP queries. Don't use OLTP queries as a yardstick when determining reasonable query timeout values for decision-support queries.
3. Double check your query timing. Not every query will be long running. You may be able to improve performance by connection pooling and caching connections to the database.
4. Don't be cavalier when it comes to specifying indexes. Indexes will speed retrieval, but they also add to your overhead. In fact, the space required for indexes can exceed the space required for data. And don't forget that the time required for updating indexes will increase as the size of the data warehouse grows.
5. Don't think small. Remember to allow for big numbers and counts, particularly when using the DatabaseMetaData class. For example, the getTables() method is likely to return a lot more information for an NCR Teradata database than a local departmental database.
6. Don't think local. Remember internationalization requirements and the effect Unicode will have on dimensions. A 200-GB database can become a 400-GB database when you use Unicode. If you have international users, be prepared for Unicode space requirements and users that want to browse Unicode dimensions.
Most of the new development in OLAP uses APIs developed by Microsoft and a number of leading OLAP vendors. They developed the specification for OLE DB for OLAP, a component-level API for accessing multidimensional data sets. Microsoft also extended the ActiveX Data Object (ADO) API to support multidimensional queries. ADO operates as an object layer over OLE DB. To use OLE DB interfaces, you must program in C/C++ or another language that can use pointers. Java programmers cannot write to OLE DB interfaces, but you can use ADO properties and methods. ADO and OLE DB decision support programs also use SQL extensions known as multidimensional expressions (MDX).
Who Uses OLAP and Why?
OLAP applications span a variety of organizational functions. Finance departments use OLAP for applications such as budgeting, activity-based costing (allocations), financial performance analysis, and financial modeling. Sales analysis and forecasting are two of the OLAP applications found in sales departments. Among other applications, marketing departments use OLAP for market research analysis, sales forecasting, promotions analysis, customer analysis, and market/customer segmentation. Typical manufacturing OLAP applications include production planning and defect analysis.
Important to all of the above applications is the ability to provide managers with the information they need to make effective decisions about an organization's strategic directions. The key indicator of a successful OLAP application is its ability to provide information as needed, i.e., its ability to provide "just-in-time" information for effective decision-making. This requires more than a base level of detailed data.
Just-in-time information is computed data that usually reflects complex relationships and is often calculated on the fly. Analyzing and modeling complex relationships are practical only if response times are consistently short. In addition, because the nature of data relationships may not be known in advance, the data model must be flexible. A truly flexible data model ensures that OLAP systems can respond to changing business requirements as needed for effective decision-making.
Although OLAP applications are found in widely divergent functional areas, they all require the following key features:
· Multidimensional views of data
· Calculation-intensive capabilities
· Time intelligence
Multidimensional Views
Multidimensional views are inherently representative of an actual business model. Rarely is a business model limited to fewer than three dimensions. Managers typically look at financial data by scenario (for example, actual vs. budget), organization, line items, and time; and at sales data by product, geography, channel, and time.
A multidimensional view of data provides more than the ability to "slice and dice"; it provides the foundation for analytical processing through flexible access to information. Database design should not prejudice which operations can be performed on a dimension or how rapidly those operations are performed. Managers must be able to analyze data across any dimension, at any level of aggregation, with equal functionality and ease. OLAP software should support these views of data in a natural and responsive fashion, insulating users of the information from complex query syntax. After all, managers should not have to understand complex table layouts, elaborate table joins, and summary tables.
Whether a request is for the weekly sales of a product across all geographical areas or the year-to-date sales in a city across all products, an OLAP system must have consistent response times. Managers should not be penalized for the complexity of their queries in either the effort required to form a query or the amount of time required to receive an answer.
The OLAP Council APB-1 performance benchmark tests a server's ability to provide a multidimensional view of data by requiring queries of varying complexity and scope. Basic aggregation is performed on some of the dimensions (product, customer, and channel). More complex calculations are performed on other dimensions. The measure dimension computes ratios and averages. Variances are computed along the scenario dimension. A complex model based on historical performance is used to compute the forecast scenario. Consistently quick response times to these kinds of queries are key to establishing a server's ability to provide multidimensional views of information.
Complex Calculations
The real test of an OLAP database is its ability to perform complex calculations. OLAP databases must be able to do more than simple aggregation. While aggregation along a hierarchy is important, there is more to analysis than simple data roll-ups. Examples of more complex calculations include share calculations (percentage of total) and allocations (which use hierarchies from a top-down perspective).
Key performance indicators often require involved algebraic equations. Sales forecasting uses trend algorithms such as moving averages and percentage growth. Analyzing the sales and promotions of a given company and its competitors requires modeling complex relationships among the players. The real world is complicated -- the ability to model complex relationships is key in analytical processing applications.
OLAP software must provide a rich tool kit of powerful yet succinct computational methods. To make developers more efficient and business users more self-sufficient, the vehicle for implementing computational methods should be clear and non-procedural. If the method for creating the desired calculations is not clear, development time and/or usage will suffer. If the calculation method is procedural, changes to the system cannot be done in a timely manner, effectively eliminating access to just-in-time information.
Whereas transaction-processing systems are judged on their ability to collect and manage data, analytical processing systems are judged on their ability to create information from data. The OLAP Council APB-1 performance benchmark contains a representative selection of calculations, both simple and complex. An example of a simple calculation contained in the performance benchmark is the calculation of margin (sales minus costs). The computation of the forecast is the most complex calculation contained in the current version of the performance benchmark. Historical data is used to project the future and aggregate data is used to estimate input data. Other more complex calculations, such as allocations and trend analysis, are also often found in OLAP systems.
Time Intelligence
Time is an integral component of almost any analytical application. Time is a unique dimension because it is sequential in character (January always comes before February). True OLAP systems understand the sequential nature of time. Business performance is almost always judged over time, for example, this month vs. last month, this month vs. the same month last year.
The time hierarchy is not always used in the same manner as other hierarchies. For example, a manager might ask to see the sales for May or the sales for the first five months of 1995. The same manager might also ask to see the sales for blue shirts but would never ask to see the sales for the first five shirts. Concepts such as year-to-date and period over period comparisons must be easily defined in an OLAP system.
In addition, OLAP systems must understand the concept of balances over time. For example, if a company sold 10 shirts in January, five shirts in February, and 10 shirts in March, then the total balance sold for the quarter would be 25 shirts. If, on the other hand, a company had a head count of 10 employees in January, only five employees in February, and 10 employees again in March, what was the company's employee head count for the quarter? Most companies would use an average balance. In the case of cash, most companies use an ending balance.
OLAP Benefits
Successful OLAP applications increase the productivity of business managers, developers, and whole organizations. The inherent flexibility of OLAP systems means business users of OLAP applications can become more self-sufficient. Managers are no longer dependent on IT to make schema changes, to create joins, or worse. Perhaps more importantly, OLAP enables managers to model problems that would be impossible using less flexible systems with lengthy and inconsistent response times, more control and timely access to strategic information equal more effective decision-making.
IT developers also benefit from using the right OLAP software. Although it is possible to build an OLAP system using software designed for transaction processing or data collection, it is certainly not a very efficient use of developer time. By using software specifically designed for OLAP, developers can deliver applications to business users faster, providing better service. Faster delivery of applications also reduces the applications backlog.
OLAP reduces the applications backlog still further by making business users self-sufficient enough to build their own models. However, unlike standalone departmental applications running on PC networks, OLAP applications are dependent on Data Warehouses and transaction processing systems to refresh their source level data. As a result, IT gains more self-sufficient users without relinquishing control over the integrity of the data.
IT also realizes more efficient operations through OLAP. By using software designed for OLAP, IT reduces the query drag and network traffic on transaction systems or the Data Warehouse.
Lastly, by providing the ability to model real business problems and a more efficient use of people resources, OLAP enables the organization as a whole to respond more quickly to market demands. Market responsiveness, in turn, often yields improved revenue and profitability.
Thanks
Saulat
No comments:
Post a Comment